Abstract
This article will review the key challenges in manufacturing today and explore the transformation that is taking place. This transformation is being driven by a new focus on resource-aware manufacturing, enabled by new technologies and capabilities including artificial intelligence, decentralized control, hybrid networking, and software-defined automation all combined to enable the digital factory of the future.
Manufacturing Challenges
Manufacturing is going through a period of transformation driven by changes in consumer demand for more personalized products and reshoring post-pandemic supply chain crunch, to name just a couple of these challenges. At the same time, governments around the world are increasing regulations to reduce carbon emissions from manufacturing to meet their net zero greenhouse gas emissions targets. Navigating these challenges will create new opportunities for industrial manufacturing companies to embrace new technologies accelerating manufacturing productivity, scalability, and flexibility while lowering carbon emissions.
In today’s brownfield manufacturing sites, there are challenges with interoperability of manufacturing and automaton equipment that have been deployed and expanded many times over the years. This has led to interoperability challenges and limited connectivity from one machine to another. Often there is no single unified network across the factory that connects all automation equipment.
To support increasing new product stock-keeping units (SKUs) requires increased setup and validation time of manufacturing production lines. For medical device manufacturing, this validation time is very expensive and time consuming. An increase in product SKUs also lowers operating equipment effectiveness (OEE) as manufacturing time and productivity is lost due to increased setup and validation time to support new product SKUs. Other manufacturing challenges include the lack of skilled workers in manufacturing. By 2030, the manufacturing industry is expected to face a shortage of approximately 2.1 million skilled workers.1 With most manufacturing taking place today in brownfield sites, this issue leads to manufacturing capacity constraints, as these manufacturing sites look to increase manufacturing output in the existing building footprint. The digital factory of the future goal is to solve these challenges and deliver the next generation of manufacturing (Figure 1).
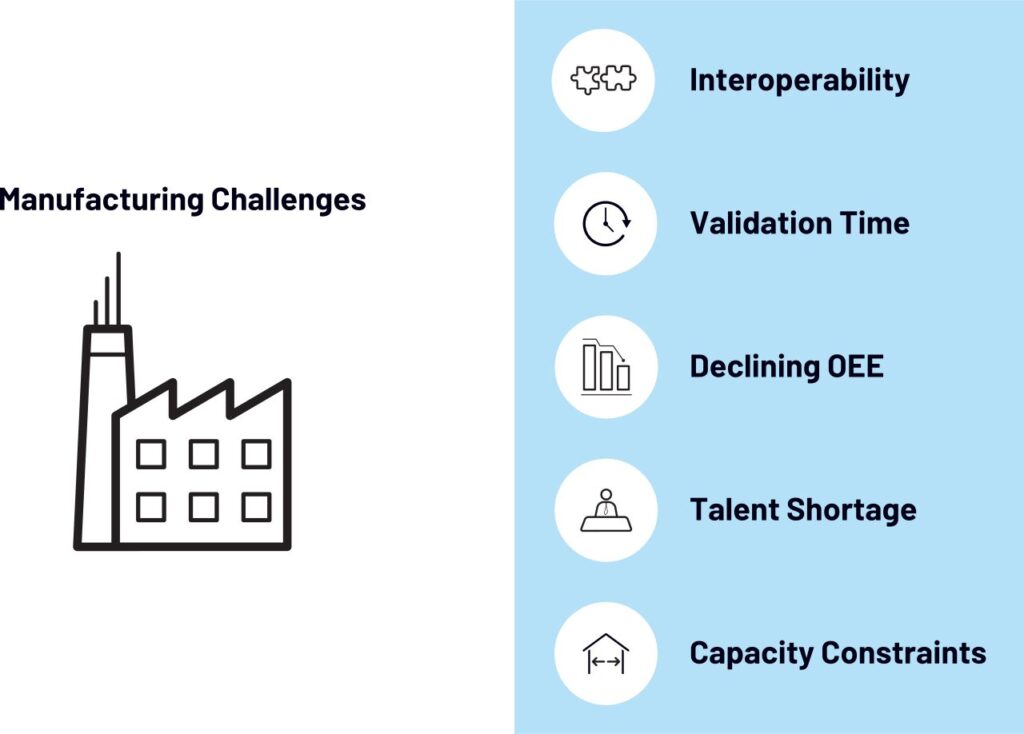
Transformation of Industrial Manufacturing
From a technological perspective, there have been significant advancements that are transforming manufacturing. Examples include sensor fusion from increased deployment of sensors on manufacturing assets and equipment to generate rich datasets that can then be used to optimize machines and increase OEE. Software-defined automation is being deployed to increase levels of manufacturing productivity, flexibility, and scalability, enabling faster setup and validation time. Finally, artificial intelligence (AI) is moving toward the edge (close to the sensor or actuator), where the data is being generated. Edge AI will transform manufacturing data into actionable insights through data-driven decision making that will enable autonomous manufacturing to unlock a step change in manufacturing productivity and competitiveness (Figure 2).
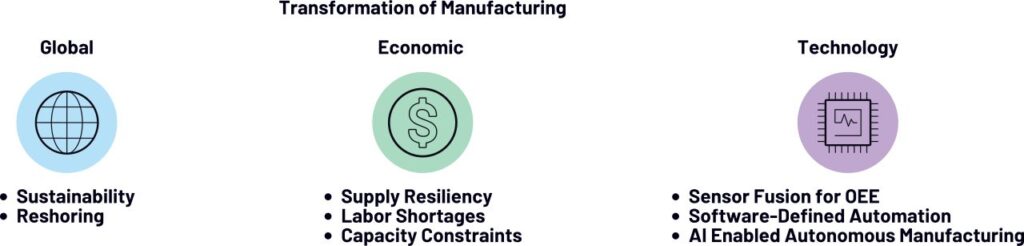
Resource-Aware Manufacturing
Next-generation manufacturing needs to look more holistically at all areas of resource consumption. The four key resources required in manufacturing are capital, power, material, and human. The digital factory of the future needs to improve the efficiency of all these resources, in the context of resource-aware manufacturing. With capital efficiency, all manufacturing capital expenditures need to show a return on investment (ROI). This may be a 1-, 3-, or 5-year ROI. One of the key goals of the digital factory of the future is to maximize profits from minimal capital expenditure to deliver the highest ROI. The second is power efficiency—next-generation manufacturing must deliver more output with less energy consumption to meet the goals of reducing global carbon emissions. Key to reducing power consumption is the deployment of higher efficiency motor drives, replacement of pneumatic actuation with electromechanical actuation, and adaptive closed-loop control to increase manufacturing efficiency.
The third area for resource-aware manufacturing is material efficiency. Reducing material waste plays a critical role in improving sustainability in manufacturing along with reduced energy consumption. By minimizing raw material usage combined with increased manufacturing, quality controls will significantly reduce material waste throughout the manufacturing flow, with a goal of zero reject production. The final area, and one of the most important, is human efficiency, with the current challenges around hiring skilled workers into manufacturing roles. Human intervention in manufacturing must be minimized where possible through more autonomous manufacturing and advanced robotics, along with automation solutions that are real-time aware and can quickly adapt to the changes in the operating environment and manufacturing requirements (Figure 3).
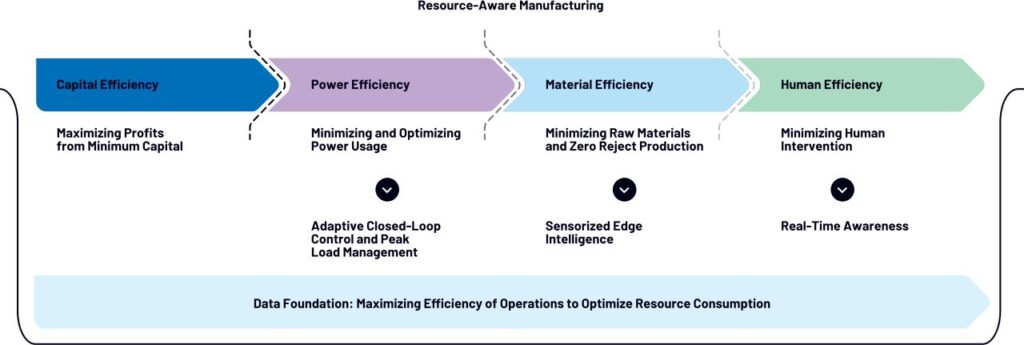
Digital Factory of the Future
Analog Devices’ vision for the digital factory of the future is centered on three key pillars: connect, control, and interpret. The connect strategy aims to deliver the promise of the factory of the future by accelerating manufacturing productivity, scalability, and flexibility while lowering carbon emissions. Ensure all manufacturing assets and machines are connected to a unified network to enable transparent access of manufacturing data. This data is then used to drive continuous process improvements across the manufacturing site. A manufacturing environment must have real-time seamless edge-to-cloud connectivity enabled through a hybrid wired and wireless factory network. For the wired control connectivity, gigabit industrial Ethernet is being deployed for higher bandwidth factory networks with time sensitive networking (TSN) for determinism of real-time traffic control. This is being complemented by flexible private 5G networks for mobile applications such as autonomous mobile robots (AMRs) and to connect to remote sensors and actuators that cannot easily be connected to a wired Industrial Ethernet network.
The second key strategy is focused on control. Decentralized autonomous control provides more flexibility through new modular automation solutions that will support increasing new product SKUs with reduced setup and validation time. By moving away from the traditional centralized programmable logic controller (PLC) of a manufacturing line to a decentralized PLC control, advanced edge computing is integrated directly into the machines. Edge-based autonomous control increases manufacturing flexibility through more reconfigurable production lines, as each machine now becomes a complete, self-contained modular manufacturing block that can be easily configured and re-deployed with significantly less human intervention. Through the increased deployment of flexible, modular manufacturing solutions, enabled through decentralized autonomous control, we can better support the goals of the digital factory of the future.
The final strategy is focused on interpret. The interpret strategy is about transforming manufacturing data into actionable insights to enable the goals of the factory of the future. Manufacturing is estimated to generate approximately 1,812 petabytes (PB) of data every year2 and the interpret strategy uses AI to transform this manufacturing data to drive productivity improvements. Key to the interpret strategy is the deployment of AI at the edge (where the data is generated). Edge AI will enable autonomous optimization in manufacturing through proactive decision making combined with sensor fusion from real-world measurement modalities (industrial vision, temperature, pressure/force, inclinometer, position, vibration, humidity, etc.). Edge AI will reduce the dependency on skilled labor by automating routine tasks and enabling more personalized and complex manufacturing with the highest yields. Key applications include guided actuation (mobile robots), defect or anomaly detection (machine health), continuous process improvements, pattern recognition (quality control), and, finally, as part of the automation control loop.
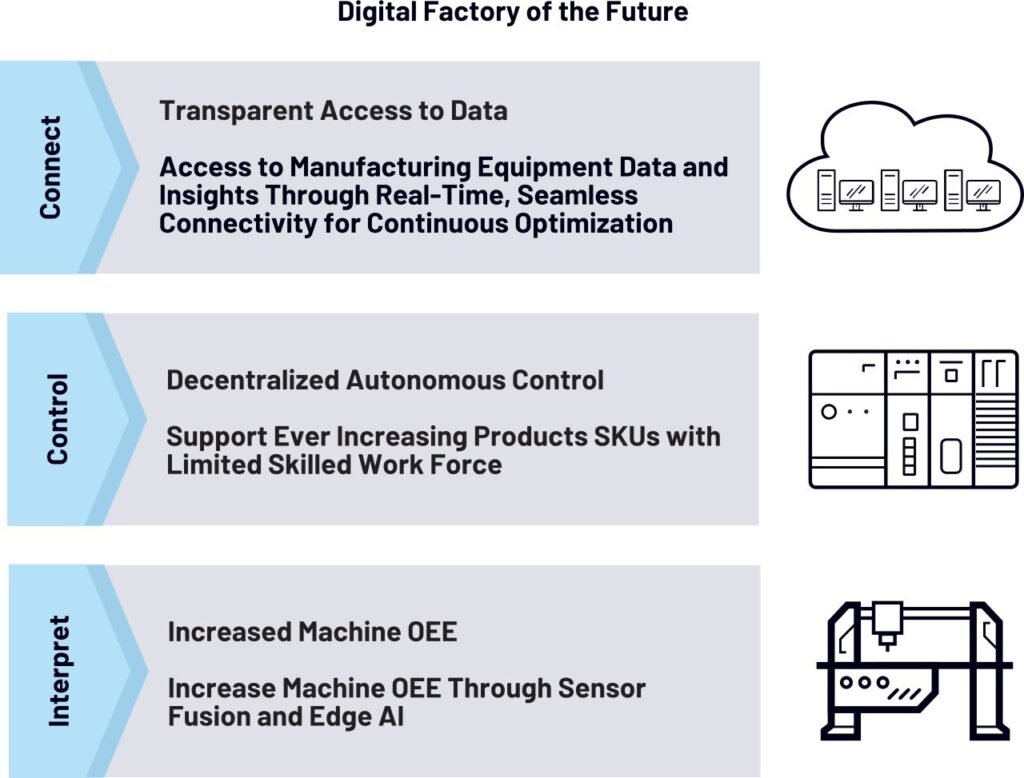
Conclusion
Manufacturing is undergoing a transformation to become smarter, more connected, and software defined. Real-time seamless edge-to-cloud connectivity is enabling transparent access to new manufacturing datasets. Decentralized control is moving the control function away from the PLC to the machine through edge computing. Sensor fusion is being deployed to increase machine OEE and create rich datasets that can be used to train and deploy AI models. Edge AI will transform automation machines to be fully autonomous. This amalgamation of new technologies is set to revolutionize the digital factory of the future, offering enhanced manufacturing productivity, flexibility, and scalability while significantly reducing energy consumption and material waste. Key for manufacturers’ success will be how they partner with companies within the ecosystem, as a variety of experience and capabilities will be important for accelerating the digital factory of the future. To learn more about ADI’s sustainable automation solutions for the digital factory of the future, visit analog.com/industrialautomation.
References
1 Victor Reyes, Heather Ashton, and Chad Moutray. “Creating Pathways for Tomorrow’s Workforce Today: Beyond Reskilling in Manufacturing.” Deloitte Insights, Manufacturing Institute, May 2021.
2 “Deloitte Survey on AI Adoption in Manufacturing.” Deloitte, 2020.
About the Author
Maurice O’Brien is the director of strategic marketing for sustainable automation at Analog Devices. He is responsible for delivering industrial automation-focused, system-level solutions. Prior to this role, Maurice spent three years working on Industrial Ethernet and 15 years working in applications and marketing roles in power management with ADI. He has a B.Eng. in electronic engineering from the University of Limerick, Ireland.